Theorem 1
Assume that the input data follows the ICA data model in (
2),
and that
G is a sufficiently smooth even function.
Then the set of local maxima
of

under the constraint
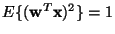
,
includes the
i-th row of the inverse of the mixing matrix

such that the corresponding independent component
si fulfills
![\begin{displaymath}E\{s_i g(s_i) - g'(s_i)\} [E\{G(s_i)\} - E\{G( \nu)\}] > 0
\end{displaymath}](img30.gif) |
(9) |
where
g(.) is the derivative of
G(.), and

is a standardized
Gaussian variable.