Next: Applications of ICA
Up: Independent Component Analysis
Previous: Identifiability of the ICA
ICA is closely related to several of the methods described
in Section 2.
- 1.
- By definition, ICA can be considered a method for achieving
redundancy reduction. Indeed, there is experimental evidence that for
certain kinds of sensory data, the conventional ICA algorithms do find
directions that are compatible with existing neurophysiological data,
assumed to reflect redundancy reduction
[14,58,116]. See Section 3.5.2.
- 2.
- In the noise-free case, the estimation of the ICA model means
simply finding certain 'interesting' projections, which give estimates
of the independent components. Thus ICA can be considered, at least
using Definitions 1 and 3, a special case of projection
pursuit. Indeed,
as will be explained in Section 4, the conventional criteria
used for finding the 'interesting' directions in projections pursuit
coincide essentially with the criteria used for estimating the
independent components.
- 3.
- Another close affinity can be found between ICA
and blind deconvolution (more precisely, the special case of
blind deconvolution where the original signal is i.i.d. over
time). Due to the assumption that the values of the
original signal s(t) are independent for different t, this problem
is formally closely related to the problem of independent component
analysis. Indeed, many ideas developed for
blind deconvolution can be directly applied for ICA, and vice versa. Blind
deconvolution, and especially the elegant and powerful framework
developed in [42], can thus be considered an intellectual
ancestor of ICA.
- 4.
- Comparing Eq. (10) in Definition 2 with the definition of
factor analysis in Eq. (5), the connection between factor
analysis and ICA becomes clear. Indeed, ICA may be considered
a non-Gaussian factor analysis.
The main difference is that usually in ICA, reduction of dimension is
considered only as a secondary objective, but this need not be the case.
Indeed, a simple combination of factor analysis and ICA can be
obtained using factor rotations. Above we saw that after finding the
factor subspace, a suitable rotation is usually performed. ICA could
also be conceived as such a rotation, where the criterion depends on
the higher-order statistics of the factors, instead of the structure
of the matrix
.
Such a method is roughly equivalent to the
method advocated in [71,61,84], which
consists of first reducing the dimension by PCA, and then
performing ICA without further dimension reduction.
- 5.
- Using Definition 1, the relation to principal component
analysis is also evident. Both
methods formulate a general objective function that define the
'interestingness' of a linear representation, and then maximize that
function.
A second relation between PCA and ICA is that both are related to
factor analysis, though under the contradictory assumptions of
Gaussianity and non-Gaussianity, respectively.
The affinity between PCA and ICA may be, however,
less important than the affinity between ICA and the other methods
discussed above. This is because PCA and ICA define their objective
functions in quite different ways.
PCA uses only second-order
statistics, while ICA is impossible using only second-order
statistics. PCA emphasizes dimension reduction, while ICA may reduce
the dimension, increase it or leave it unchanged.
However, the relation between ICA and nonlinear versions of the PCA criteria,
as defined in [82,112], is quite strong, as
will be seen in the next Section.
The connections between ICA and some other methods are illustrated in
Fig. 4. The lines in the diagram indicate very close
connections, under the assumptions given next to the lines.
First, if no assumptions on the data are made, and in particular no noise is
postulated in the data, ICA can be considered a method of exploratory
data analysis, as projection pursuit. Indeed, using Definition 1, ICA
means simply finding some interesting projections of the data, and the
measures of interestingness are essentially equivalent in the two
methods, as will be seen in the next Section.
On the other hand, if one assumes a noisy data model, as in Definition 2, ICA
can be considered a variation of factor analysis for
non-Gaussian data. Thus one has two possible approaches to ICA that
are quite different as they stem from two clearly distinct classical
methods.
ICA according to Definition 3, or the noise-free ICA data model, is
something between these two approaches.
As for PCA, its connection to ICA can be considered indirect, since it
can be used to perform factor analysis for Gaussian data.
Figure 4:
The relations between ICA and some other methods. The lines
show close connections, and the texts next to the lines show the
assumptions needed for the connection.
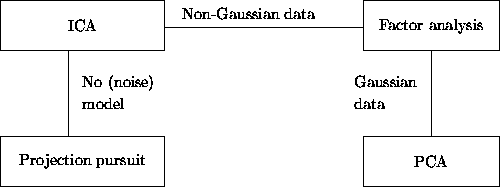 |
Next: Applications of ICA
Up: Independent Component Analysis
Previous: Identifiability of the ICA
Aapo Hyvarinen
1999-04-23